Logistics Business Intelligence and shipping data analysis: 8 tools & metrics

In the logistics industry, Business Intelligence is radically transforming the way the supply chain is managed. Indeed, logistics data analytics is enabling cost optimization, improved operational efficiency, and increased customer satisfaction through decisions based on concrete, measurable information.
In this guide, we will explore how to effectively implement shipment analysis in your company, what the tangible benefits are, and how to concretely measure the results achieved. We will also show you how large companies are using these tools to maximize productivity and reduce operating costs.
Fundamentals of Business Intelligence in Logistics
First and foremost, when we talk about data analysis in shipping, we are referring to a set of tools and techniques that allow us to transform raw data into practical information. Specifically, these systems allow us to monitor warehouse occupancy, optimize routes, and ensure more accurate order management.
The measurable benefits to businesses are significant. Through the implementation of business intelligence solutions, it is possible:
- Centralize and standardize the data available in the company
- Reduce storage costs and rationalize space
- Develop predictive scenarios based on sales history
- Improve delivery quality and safety
- Optimize warehouse flows
Types of analysis to implement
- Descriptive Analysis:This type of analysis is critical for obtaining a clear and detailed picture of the current state of a specific department or process. It uses historical data to provide a visual and quantitative representation of current activities, allowing you to understand "what happened." It is ideal for real-time performance monitoring and establishing baselines for comparison.
- Diagnostic Analysis: It follows descriptive analysis and focuses on interpreting the data to identify the causes of problems that have arisen. This approach is crucial to understand the reasons behind certain performance, whether positive or negative, and to isolate the factors that influence results. The goal is to identify effective solutions and intervention strategies to address the trouble spots.
- Predictive Analysis:Through the use of statistical models and machine learning algorithms, predictive analytics examines existing data patterns to predict future events or behaviors. This type of analysis is particularly useful for anticipating problems, optimizing decision making, and improving strategic planning by identifying possible scenarios and their likelihood of occurrence.
- Prescriptive Analysis: Prescriptive analysis goes beyond prediction, offering recommendations on how to act in the face of predictions made. It uses the results of predictive analytics to formulate action plans that enable companies to prevent or mitigate future risks. This analysis supports strategic and operational decision-making, guiding organizations in proactively managing medium- and long-term challenges.
These types of analysis, if implemented correctly, can transform the way a company understands and reacts to its operating environment, leading to more informed and strategic management of resources.
Practical implementation of shipment analysis
Constructing a system for data collection and analysis can vary depending on the complexity of each individual situation. There are applications that, once integrated into one's technology stack, act autonomously by providing detailed tables and reports. In other cases, a more structured process is required, such as the following.
Step 1: Data collection and metrics definition
In order to implement an effective shipment analysis system, we need to start from the ground up. Business Intelligence in logistics first requires structured data collection from various sources such as GPS trackers, sensors, and RFID tags. This allows us to gain a comprehensive view of our logistics operations.
In the initial setup, it is critical to establish a clear and concise reporting system. Through the use of the appropriate BI tools, we can monitor and optimize operations in real time, clearly identifying opportunities for improvement.
As for the KPIs to be monitored, these can change depending on the needs of each individual company. Among the many metrics we have for example:
- Delivery Time: the time it takes to deliver a product to the customer
- Transport Cost per Unit: the total transportation cost divided by units shipped
- On-time Delivery Rate: percentage of on-time deliveries
Step 2: Establishment of an operations center for analysis
To ensure that the data collected is not only present but also actively used for business improvement, it is crucial to make it accessible and understandable to all levels of the organization.
The creation of a specialized statistics analysis team is the first step to the democratization of data access. This team will be tasked with processing and interpreting information so that even those without specific skills in data analysis or statistics can understand and use it effectively.
This accessibility opens the opportunity for all members of the company to contribute to growth and continuous improvement. For example, by implementing predictive analytics, models can be used to anticipate demand and optimize inventory and shipping routes. Advanced analytics can proactively predict and mitigate potential delays caused by adverse weather conditions, traffic problems, or mechanical failures, thus avoiding negative impacts on service.
In addition, the integration of the Business Intelligence (BI) system with other business platforms, such as CRM, ERP, and IoT solutions, is essential to ensure a holistic and integrated view of operations. This integration improves the accuracy of strategic decisions, enabling more effective resource management and faster response to market needs.
Democratizing access to data through a specialized team, leveraging predictive analytics, and integrating IT systems are key steps in transforming data into valuable tools for the entire enterprise, promoting an inclusive and informed work environment where every contribution is directed toward the common goal of business growth.
Tools for shipping data analysis: 8 examples
Tableau
Tableau is a powerful data visualization tool that enables companies to transform large amounts of information into intuitive charts and interactive dashboards. Specifically for shipment analysis, Tableau can help track metrics such as delivery times, shipping costs, and on-time delivery rates. Users can easily explore data to identify trends, patterns, and anomalies, thereby improving operational and strategic decisions.
Microsoft Power BI
Microsoft Power BI is a business analysis tool that facilitates the creation of detailed reports and customizable dashboards. It offers drill-down capabilities that are particularly useful for logistics analysis, allowing users to break down datasets to uncover crucial details about shipping operations. Power BI easily integrates data from multiple sources, improving the effectiveness of forecasting and logistics decisions.
Qlik Sense
Qlik Sense is a data analytics platform that features an intuitive interface and the ability to integrate data from multiple sources. For shipping, Qlik Sense can be used to effectively analyze workflows, optimize routes, and predict future capacity needs, helping to reduce costs and improve efficiency.
Google Analytics
Google Analytics, although primarily a web analytics tool, can be effectively used to track and analyze user behaviors related to online orders and deliveries. Through the configuration of specific events and targets, companies can gain valuable insights into the performance of their e-commerce and logistics operations.
SAS Analytics
SAS Analytics offers advanced analytics solutions that can also be applied to the shipping industry. Through the use of statistical and analytical models, SAS helps companies forecast shipping demand, optimize routes, and manage risks and uncertainties, improving planning and operational efficiency.
IBM Cognos Analytics
IBM Cognos Analytics is a BI tool that enables advanced reporting and predictive analytics. For the shipping industry, Cognos can facilitate logistics performance management by providing detailed analysis of cost, delivery time, and customer satisfaction, supporting more informed and timely decisions.
Splunk
Splunk is software that enables the collection and analysis of large amounts of machine-generated data, including logistics data. It can be used to monitor real-time shipping conditions, analyze shipping logs, and predict potential problems before they affect operations, thus providing a comprehensive and proactive view of shipment management.
Bonus: ShippyPro Optimizer
One new ShippyPro feature is Optimizer, a Shipping Intelligence tool to track key shipping KPIs. All available data can be filtered by Country and Courier so you get a clear snapshot of shipping costs anywhere in the world.
Optimizer is available to all Professional ShippyPro users and you can expand the available reports with custom add-ons.
Conclusion: business intelligence and shipment analysis
Data analysis in modern logistics is a strategic necessity, no longer an option.
True examples demonstrate how shipment analytics achieves tangible results: reduced operational costs, route optimization, improved warehouse management, and increased customer satisfaction. These benefits translate directly into competitive advantages that can be measured through specific KPIs.
The key to success lies in adopting appropriate tools and implementing structured processes.
So, as the logistics industry continues its digital evolution, companies that make the most of data analytics will position themselves as market leaders. The important thing is to get started now, starting with clear and measurable objectives, to gradually build a data-driven management system that guarantees concrete and lasting results.
Logistics data analysis FAQs
What are the main benefits of data analysis in shipping?
Data analysis in shipping enables you to optimize costs, improve operational efficiency, reduce waste, and increase customer satisfaction through decisions based on factual and measurable information.
How do you implement an effective shipment analytics system?
An effective shipment analytics system requires structured data collection from multiple sources, use of appropriate BI tools, monitoring of key metrics such as delivery time and transportation cost per unit, and implementation of operational best practices such as predictive analytics.
What are the key KPIs to track in logistics data analysis?
The most significant KPIs include picking and warehousing efficiency, inventory management, lead times, accuracy of filled orders, and operating costs per unit. These indicators are essential for measuring the success of logistics operations.
How can costs be optimized through shipment analysis?
Cost optimization is achieved through detailed analysis of transportation costs, route optimization using advanced software, and reduction of operational waste through predictive analytics and automation of processes such as label creation and courier selection.
What is the difference between Business Intelligence and Business Analytics in logistics?
Business Intelligence focuses on descriptive analysis, providing insight into historical and current data, while Business Analytics focuses on predictive analysis, using techniques such as data mining and machine learning to predict future trends and optimize logistics operations.
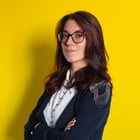
Curious by nature, analytical by mindset, Giulia Castagna is the voice behind ShippyPro’s content. As Content Marketing Manager, she simplifies complex logistics topics for those who ship around the world every day. She writes about AI, automation, and shipping trends to inspire data-driven decisions.